UXR Needs a Seat at the AI Table (Even If It's the Folding Chair from the Break Room)
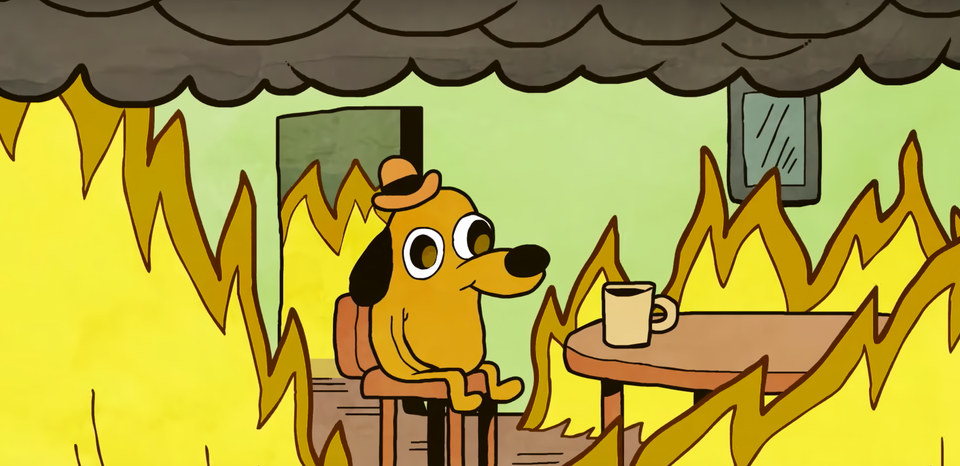
If archeologists of the future excavate the ruins of today's AI industry, they'll uncover a peculiar artifact: the complete absence of evidence that humans were ever consulted about products built explicitly for them. Like discovering a civilization that manufactured shoes without ever measuring a foot. In Silicon Valley's grand narrative of AI, one character is conspicuously missing: the human being.
Welcome to the AI industry in 2025.
Every morning, thousands of engineers wake up, brush their teeth, and head to work to build tools for creatures they've never systematically studied. Their Slack channels overflow with discussions about transformer architectures and attention mechanisms without a single thread dedicated to how actual humans process information or make decisions.
The industry's organizational charts tell the story more clearly than any manifesto could. At one major AI lab, a colleague counted 157 machine learning engineers, 32 data scientists, 28 infrastructure specialists... and a single user researcher. When I asked an executive about this, he beamed with pride: "We're very committed to understanding our users. Julie does surveys every quarter!"
AI companies plaster "human-centered" across keynotes and investor decks like nutritional claims on sugary cereals. It's technical jargon masquerading as ethical commitment—impressive-sounding words that suggest someone, somewhere has consulted a human who isn't on the engineering team.
The reality? User research in AI development exists primarily as a theoretical concept—something acknowledged in principle but neglected in practice, like New Year's resolutions or reading the terms of service.
AI labs aren't temples of misanthropy—they don't actively hate humans so much as find them irrelevant to the technical challenges at hand. The typical development process relies on a peculiar form of empathy: engineers imagine what they themselves might want, multiply by several billion people, and call it "user understanding."
The resulting disconnect is hardly subtle. When a senior researcher recently boasted that their new chatbot was "deeply intuitive," I asked how many usability studies they'd conducted. The awkward pause was followed by: "Well, everyone on the team found it easy to use."
For those unfamiliar with how AI products come to market, allow me to pull back the curtain on this peculiar theater of technological hubris:
Inception: A PhD or three decide that a particular problem could benefit from more matrix multiplication.
Development: Engineers build models that perform impressively on data that bears only passing resemblance to messy reality.
Optimization: Benchmarks are chased with the fervor of religious devotees. Numbers go up. Champagne is poured.
Integration: The model is wedged awkwardly into a product shaped entirely by engineering constraints and executive fantasies.
Launch: The world is promised nothing short of digital divinity.
Reality: Users stare in confusion at interfaces designed by people who have never watched another human use a computer.
Denial: "The users just don't understand how revolutionary this is."
Rationalization: "We need to educate users on the proper way to use our perfectly designed system."
Iteration: Return to step 2, having learned nothing about humans but much about how to make more compelling demo videos.
At no point in this cycle is there a dedicated moment for understanding how people might actually use, misuse, or be confused by the technology. The assumption—never stated but always present—is that sufficient intelligence will somehow bypass the need for usability. As if the history of technology isn't littered with brilliant systems that failed because humans couldn't figure out how to use them. Like that voice-controlled blender that confidently liquefied your phone when you said "I need to call mom."
Perhaps the most remarkable achievement of the AI industry is its ability to use the phrase "human-centered AI" with completely straight faces in boardrooms where no actual user research has ever been presented. It's linguistic sorcery of the highest order, conjuring the impression of empathy from the raw materials of indifference. The term "human-centered" in AI product meetings functions much like "organic" on food packaging—a label that means nothing while suggesting everything.
What passes for user research in AI development would make any seasoned UX professional weep into their carefully crafted research plans:
"We showed it to some people in the office, and they thought it was cool."
"Our beta testers haven't complained too loudly."
"I used it myself and it seemed fine."
"The engagement metrics aren't terrible."
"My mom didn't immediately hate it, and she's technologically challenged."
"We did a survey and 78% of respondents agreed it was 'very innovative' – mostly after we explained what it actually does."
These are not exaggerations. These are actual justifications I've heard for shipping AI products to millions of users, products that will influence decisions, shape information access, and potentially alter the trajectory of human lives.
The lack of user research manifests most visibly in AI interfaces, which have developed their own distinctive aesthetic—created entirely without investigating how humans actually process information or make decisions. These interfaces present a curious blend of minimalism and opacity that serves primarily to hide the fact that nobody knows exactly what the system is doing. Clean white backgrounds. Pulsing dots to indicate "thinking." Single text fields that offer no clue about what you might ask or how you might ask it. This design approach isn't accidental—it's the inevitable result of never watching real humans interact with your product, like writing a cookbook without ever tasting food.
Take agentic AI, a perfect case study in how not to design an interface for a system that's supposedly making decisions for you. The UI presents a bewildering array of actions being taken, code being generated, and decisions being made, all with the explanatory clarity of a fever dream. Commands appear and disappear without context. System status is communicated through cryptic messages that read like technical logs rather than human-facing information. The entire experience feels like watching someone else use your computer while you try to deduce what they're doing from sporadic over-the-shoulder glances. It's the technological equivalent of letting a hyperactive toddler reorganize your kitchen while you're blindfolded—you know something's happening, but you've lost all agency in the process.
The entire category of agentic AI interfaces seems to have adopted a design philosophy best described as "mystery theater." They proudly violate every principle of usability that's been established over the past forty years:
User understanding of capabilities? Never systematically studied, forcing users to play a frustrating guessing game about what the system can actually do.
Mental models? No research conducted on how users conceptualize AI systems, leading to profound mismatches between expectations and reality.
Information needs? Never identified through actual observation, leaving users starved for context and explanation when they need it most.
Usage patterns? Completely uninvestigated, resulting in workflows that fight against how humans naturally approach tasks.
Error recovery strategies? Left unexplored, with no understanding of how users actually try to correct mistakes when they occur.
Learning curve? Unstudied, creating systems that are unnecessarily difficult to master because no one observed the progressive stages of user adoption.
Trust calibration? Ignored entirely, resulting in either blind faith in AI outputs or complete rejection, with no research into how to help users appropriately gauge system reliability.
These aren't merely interface failures but evidence of a complete absence of user research—fundamental betrayals of the premise that these tools should make complex tasks simpler. Without studying how humans use AI, companies have made the simple task of understanding what a computer is doing on your behalf nearly impossible.
These aren't mere oversights. They're research voids—vast empty spaces where systematic user studies should have generated insights but instead left only the barren landscape of engineering assumptions. Without research, everything becomes a guess, a hope, or worse—an engineer's conviction that their personal experience represents universal human behavior.
The absence of user research in AI development isn't merely an academic concern or professional slight. It has real consequences that ripple through our increasingly AI-mediated world:
- AI healthcare tools with interfaces so confusing that physicians make diagnostic errors.
- Financial AI that presents options in ways that nudge users toward poor economic decisions.
- Educational AI that reinforces misconceptions because nobody tested whether students actually understand the explanations.
- Content moderation AI that fails to account for how users will inevitably attempt to circumvent it.
- Recommendation systems that create filter bubbles because nobody studied how real humans explore information spaces.
- Legal AI that confidently cites nonexistent case law while providing no way for attorneys to verify its hallucinations.
- HR systems that automate discrimination while hiding behind algorithmic opacity.
These aren't hypotheticals. They're happening now, with real people bearing the consequences of an industry that couldn't be bothered to hire sufficient researchers to understand how their products would actually be used.
When confronted with the absence of user research in their development processes, AI companies deploy a remarkable array of defenses, each more transparent than the last:
"We move too fast for traditional research." Translation: We value speed over understanding.
"The technology is too complex for users to provide meaningful feedback." Translation: We don't know how to explain our own product.
"Our AI adapts to the user, so it doesn't need to be designed for usability." Translation: We're hoping the model will somehow compensate for our poor interface decisions.
"We do internal testing with our team." Translation: We asked some engineers who have been staring at this problem for two years whether it made sense to them.
"User research is built into our development process." Translation: We read tweets about our product after it launches.
"We're creating a new paradigm of human-computer interaction." Translation: We couldn't be bothered to learn about the old paradigm.
"Our early adopters will guide our development." Translation: We're using paying customers as unpaid research participants.
These aren't strategies. They're excuses wrapped in the language of innovation, as if understanding humans were somehow an outdated concept rather than the entire point of building technology in the first place.
To be fair—though fairness seems a quaint concept in this context—some AI companies are beginning to realize that perhaps understanding users might be valuable. These rare enlightened organizations can be identified by several distinctive characteristics:
They have more than one user researcher.
They conduct research before building products, not just after launching them.
They actually change their products based on research findings.
They view user confusion as a product failure, not a user failure.
They recognize that AI interfaces require more explanation, not less.
They understand that "it works on my machine" is not a sufficient testing protocol.
They accept that their AI model doesn't automatically understand user needs just because it occasionally generates a coherent paragraph.
These companies remain the exception rather than the rule, islands of understanding in a vast sea of assumption and technical solutionism.
For those building AI who have somehow stumbled upon this article (perhaps your lone researcher forwarded it in a final act of professional desperation before returning to their folding chair in the corner of your open office), I offer these suggestions:
Hire researchers. Not one. Not two. Many. Enough that they can actually study the various contexts in which your AI will be used before you build it.
Listen to them. This step is crucial and frequently overlooked. Having researchers who are ignored is only marginally better than having none at all.
Recognize that intelligence does not equal usability. Your model's impressive benchmark scores do not translate directly to user satisfaction or effectiveness.
Understand that explanations matter. Users need to understand what your AI can do, what it can't do, what it's doing right now, and why it made the choices it did.
Test with real humans who don't work at your company. Your engineering team is not representative of your user base. Neither is your executive team. Neither is your venture capital firm.
Accept that your AI is not a mind reader. Even the most advanced models still need thoughtful interfaces to bridge the gap between silicon and consciousness.
Remember that when users struggle, blaming their "prompt engineering skills" is like blaming someone's skiing technique when you've given them a pair of toothbrushes instead of skis.
The truly remarkable thing about user research is not that it's difficult or expensive, but that it's been proven effective for decades across countless industries. The knowledge exists. The methodologies are well-established. The only missing ingredient is the will to employ them.
In the end, we don't need a throne. We don't need a corner office. We simply need a chair at the table when decisions are made—even if it's a folding chair brought from home. Because one researcher with a voice is worth more than a hundred silent observers. So the next time you see that lone UX researcher setting up their folding chair in the corner of your AI strategy meeting, maybe pull up a real seat for them instead. Your users will thank you. And if they don't, that's actually the point—they'll be too busy successfully using your product to notice the elegance of its design. Or as one AI CEO might put it: "The best user research is the research you never notice we didn't do."
🎯 Still here?
If you’ve made it this far, you probably care about users, research, and not losing your mind.
I write one longform UX essay a week — equal parts strategy, sarcasm, and survival manual.
Subscribe to get it in your inbox. No spam. No sales funnels. No inspirational LinkedIn quotes. Just real talk from the trenches.
👉 Subscribe now — before you forget or get pulled into another 87-comment Slack thread about button copy.